I’m Xiaolin Hu, pursuing Ph.D. at Gaoling School of Artificial Intelligence , Renmin University of China (RUC). I am fortunate to be advised by Prof. Yong Liu. Currently I am a Research Intern at Xiaomi’s AI Lab
, where I focus on Edge Large Language Models (LLMs), mentored by Wei Liu and Jian Luan. Previously, I received my B.E. and M.E. degrees in communication and information system from Shanghai University in 2018 and 2021, respectively. Between 2018 and 2021, I collaborated with Prof. Nicholas E. Buris as part of the Intelligent Multi-Input Multi-Output Systems (i-MIMOs) research group. Additionally, I gained research experience as an Intern at the OPPO Research Institute
from October 2020 to February 2021, under the mentorship of Xianyue Wu and Tehuang Liu.
I am currently bridging LLMs and personal edge devices. With a long-term goal of contributing to a human-centered application ecosystem based on large models, I am particularly interested in:
(1) Science-Driven LLMs Training: Exploring the scientific principles of LLMs training and fine-tuning.
(2) Personal Edge LLMs Serving: Developing efficient algorithms for LLMs on edge devices to enhance personalized services.
I won the Shanghai University President Scholarship (The highest honor among the scholarships at Shanghai University).
🔥 News
- 2024.12: Two papers are accepted by ICLR 2025!
- 2024.12: One paper is accepted by AAAI 2025! Stability and Generalization of Zeroth-Order Decentralized SGD with Changing Topology.
- 2024.11: One paper is accepted by COLING 2025! PMSS: Pretrained Matrices Skeleton Selection for LLM Fine-tuning.
- 2024.9: One paper is accepted by NeurIPS 2024! Enhancing In-Context Learning with just SVD-Based Pruning: A Theoretical Perspective.
- 2024.6: Xiaomi Young Scholar Research Program (PI: Yong Liu) received approval, focusing on fine-tuning edge LLMs for personalized services.
- 2024.5: One Paper is accepted by KDD 2024! LLMs may Dominate Information Access.
- 2023.12: Started internship at Xiaomi AI Lab, focusing on efficient fine-tuning of edge LLMs.
📝 Publications
🎙 Federated Learning Generalization
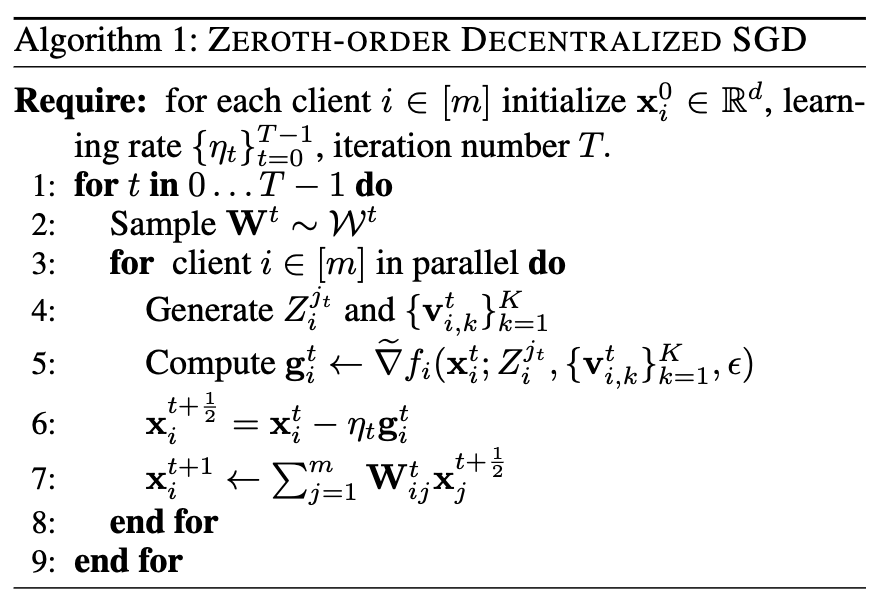
Stability and Generalization of Zeroth-Order Decentralized Stochastic Gradient Descent with Changing Topology
Xiaolin Hu, Zixuan Gong, Gengze Xu, Wei Liu, Jian Luan, Bin Wang, Yong Liu (Oral)
- This paper provides the first generalization analysis of ZO-DSGD with changing topology.
- The obtained generalization bounds align with SGD in (strongly) convex cases and with DSGD in non-convex cases.
- The results reflect the impact of client count, sample size, and topology on generalization performance.
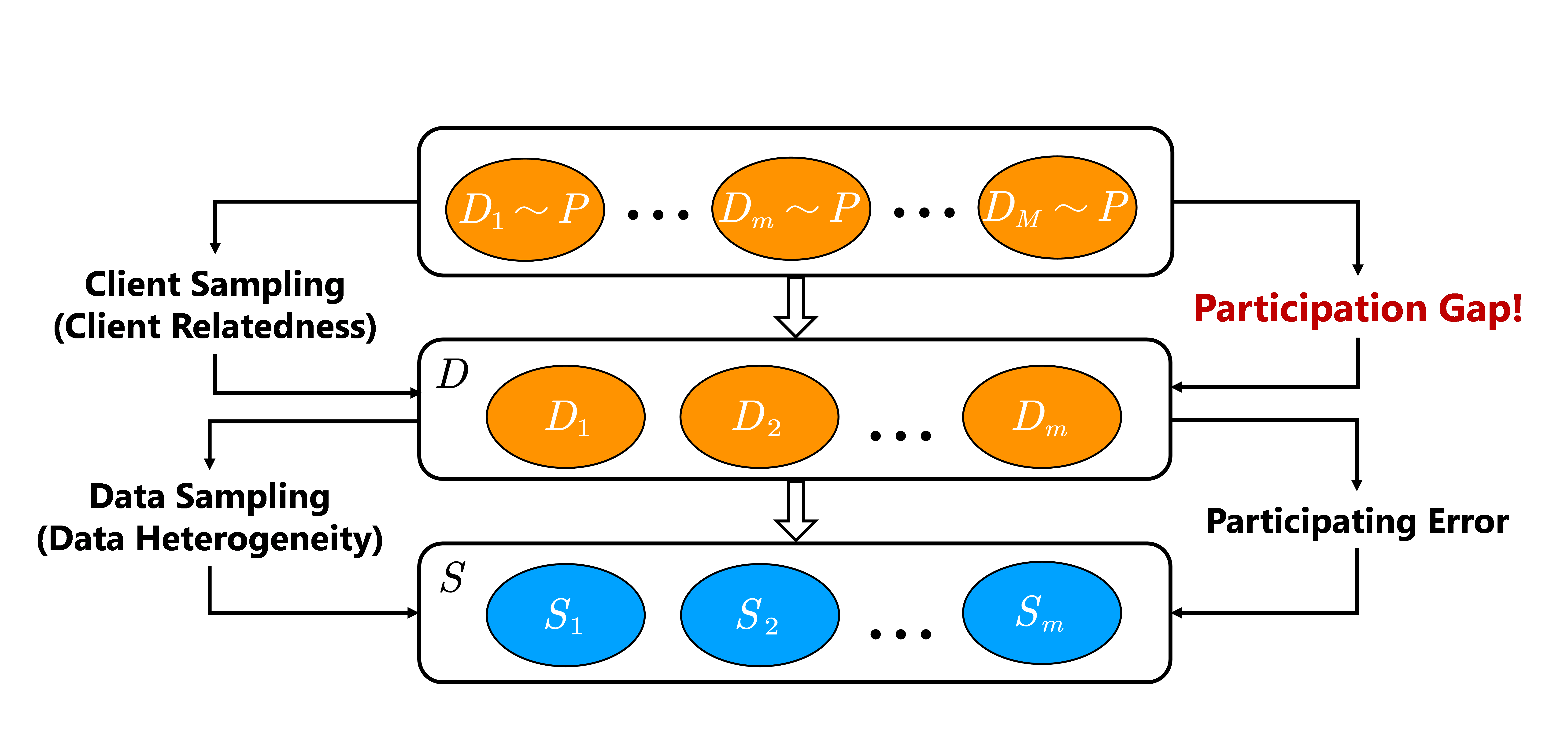
Generalization Bounds for Federated Learning: Fast Rates, Unparticipating Clients and Unbounded Losses
Xiaolin Hu, Shaojie Li, Yong Liu
- We present a theoretical analysis of the generalization error for non-participating clients in federated learning.
- The obtained generalization bounds in high probability form capture the performance of a single trial, rather than the average over multiple trials.
- We derive generalization bounds for heavy-tail losses, applicable to federated learning with unbounded losses, such as cross-entropy.
🧑🎨 Large Language Models
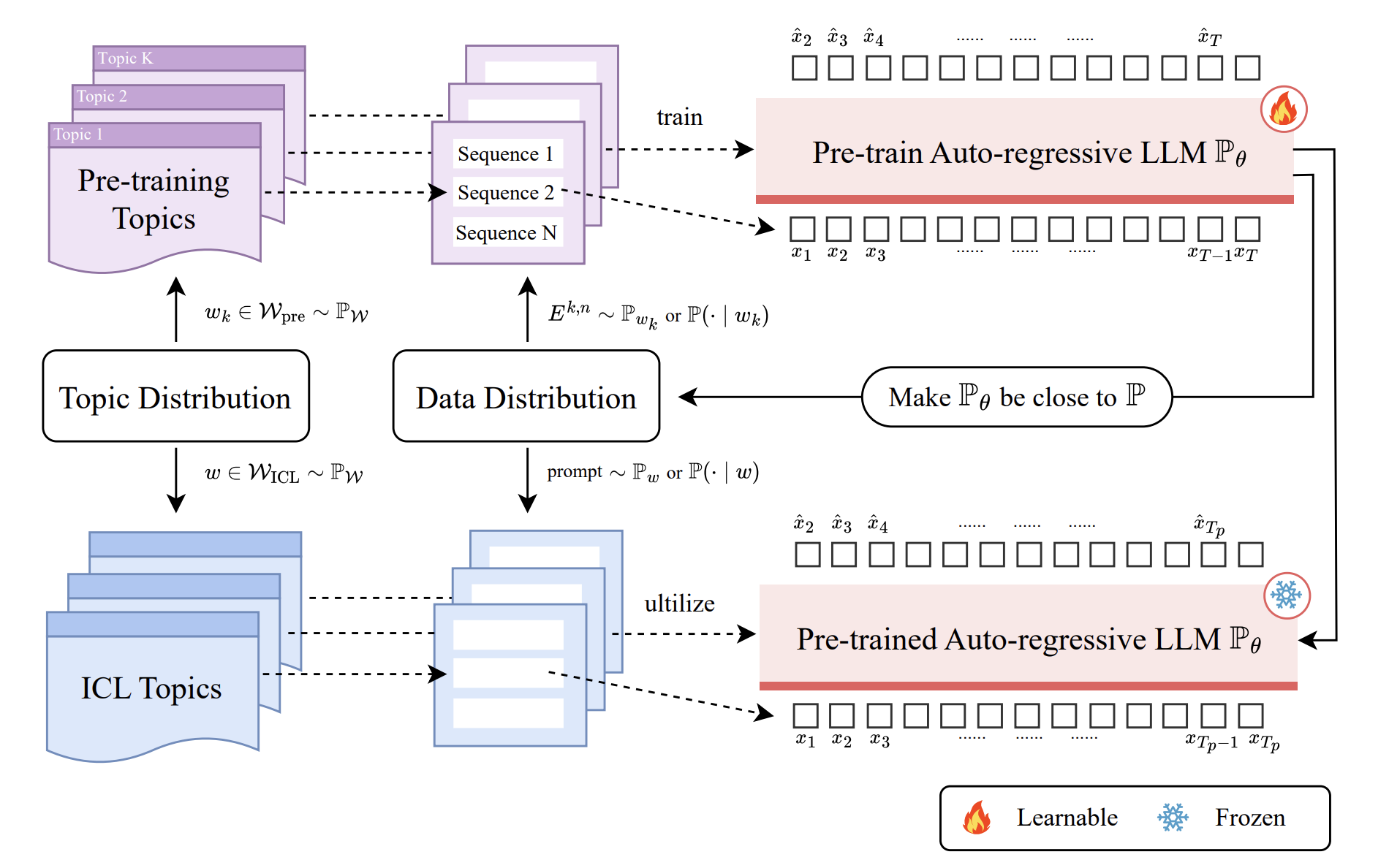
Towards Auto-Regressive Next-Token Prediction: In-context Learning Emerges from Generalization
Zixuan Gong*, Xiaolin Hu*, Huayi Tang, Yong Liu (* Equal contribution)
- We explore the emergence of in-context learning (ICL) capabilities in auto-regressive next-token prediction models.
- To bridge the pre-training and ICL phases, we introduce a two-level expectation over data and topic distributions, providing PAC-Bayes generalization bounds to support our analysis.
- Additionally, we model the training process using Stochastic Differential Equations (SDEs), demonstrating that ICL arises from the exceptional generalization across sequences and topics.
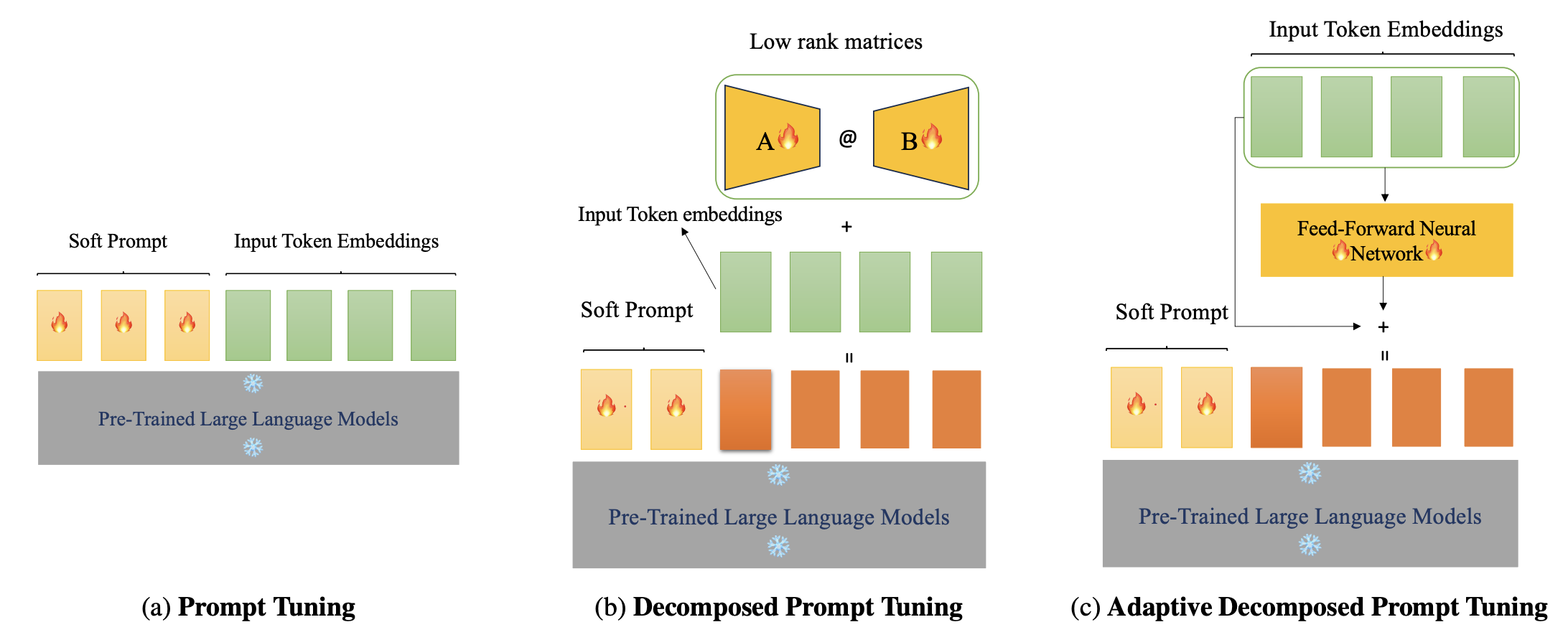
ADePT: Adaptive Decomposed Prompt Tuning for Parameter-Efficient Fine-tuning
Pengwei Tang, Xiaolin Hu, Yong Liu
- We propose Adaptive Decomposed Prompt Tuning (ADePT), which can produce unique token embedding offset for each token.
- ADePT addresses the limitations of DePT, enabling better optimization and generalization without increasing inference time or parameters.
- Experiments on 23 NLP tasks and 4 PLMs show ADePT outperforms leading PEFT methods and even full fine-tuning in some cases.
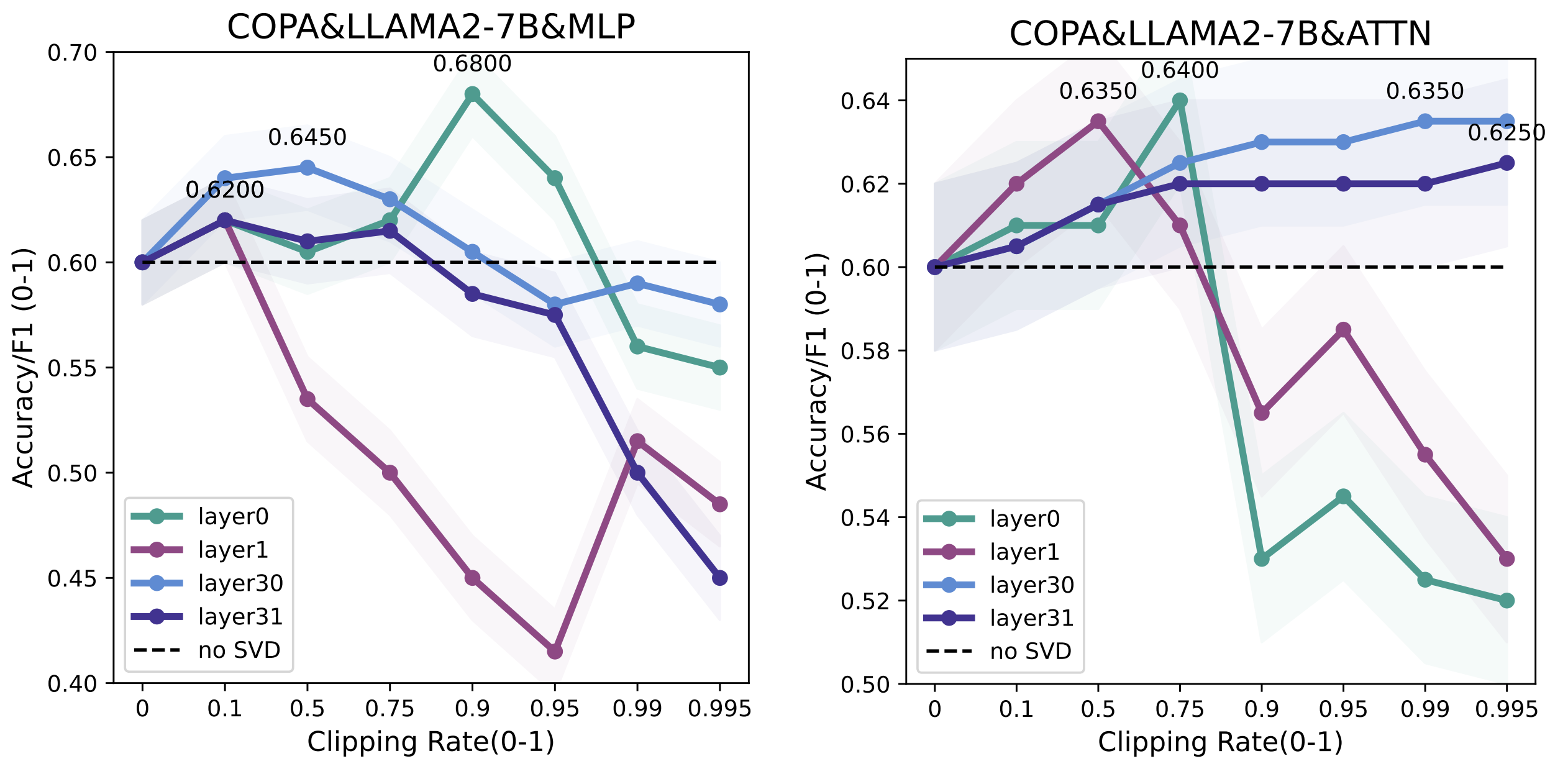
Enhancing In-Context Learning with just SVD-Based Pruning: A Theoretical Perspective
Xinhao Yao, Xiaolin Hu, Shenzhi Yang, Yong Liu
- We show an exciting phenomenon that SVD-based weight pruning can enhance In-Context Learning (ICL) performance.
- we conduct theoretical analysis by presenting the implicit gradient descent (GD) of ICL and giving generalization bounds of ICL.
- We further propose a simple, derivative-free algorithm to enhance ICL. Experiments demonstrate its effectiveness.
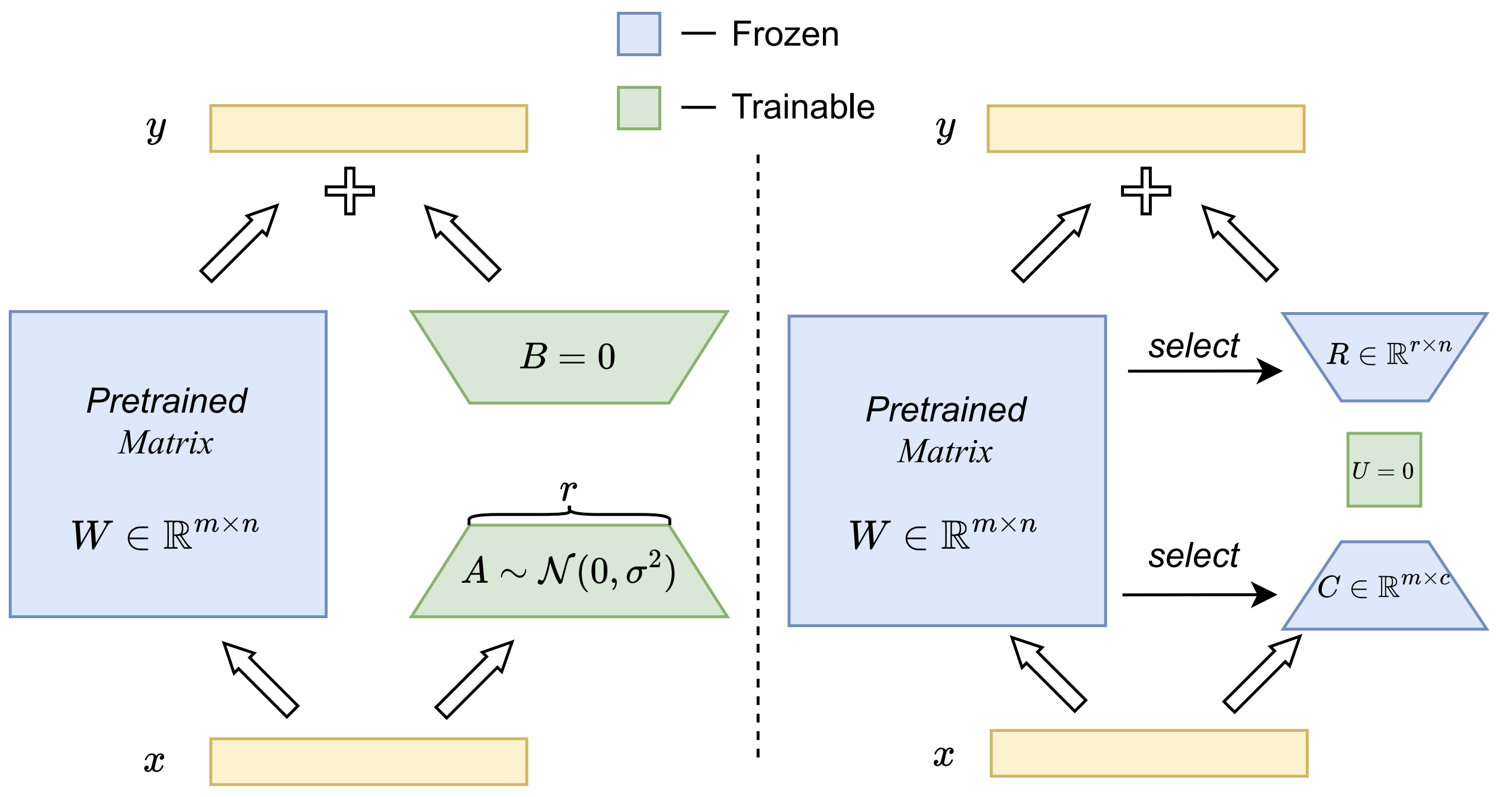
PMSS: Pretrained Matrices Skeleton Selection for LLM Fine-tuning
Qibin Wang, Xiaolin Hu, Weikai Xu, Wei Liu, Jian Luan, Bin Wang
- We propose PMSS, enabling high-rank updates at low costs by selecting skeletons from pre-trained weights.
- PMSS overcomes LoRA’s low-rank limitations and optimizes initialization to utilize semantic and linguistic information.
- Experiments show PMSS outperforms LoRA and excels in tasks like DROP and math reasoning with fewer trainable parameters.
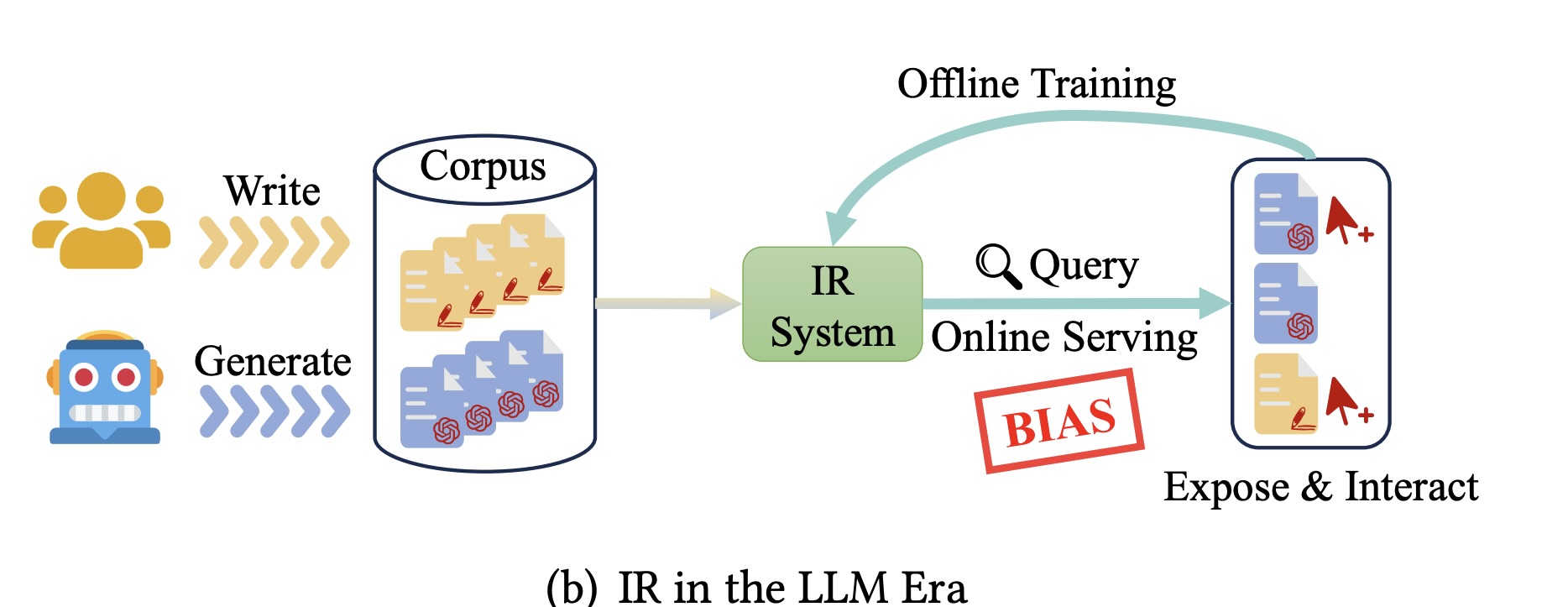
Neural Retrievers are Biased Towards LLM-Generated Content
Sunhao Dai, Yuqi Zhou, Liang Pang, Weihao Liu, Xiaolin Hu, Yong Liu, Xiao Zhang, Gang Wang, Jun Xu
- We explore how LLM-generated texts influence IR systems, revealing a source bias where neural models favor LLM-generated documents.
- We use information theory to explain this bias, showing it arises from the focused semantics of LLM-generated content.
🧬 AI+Science
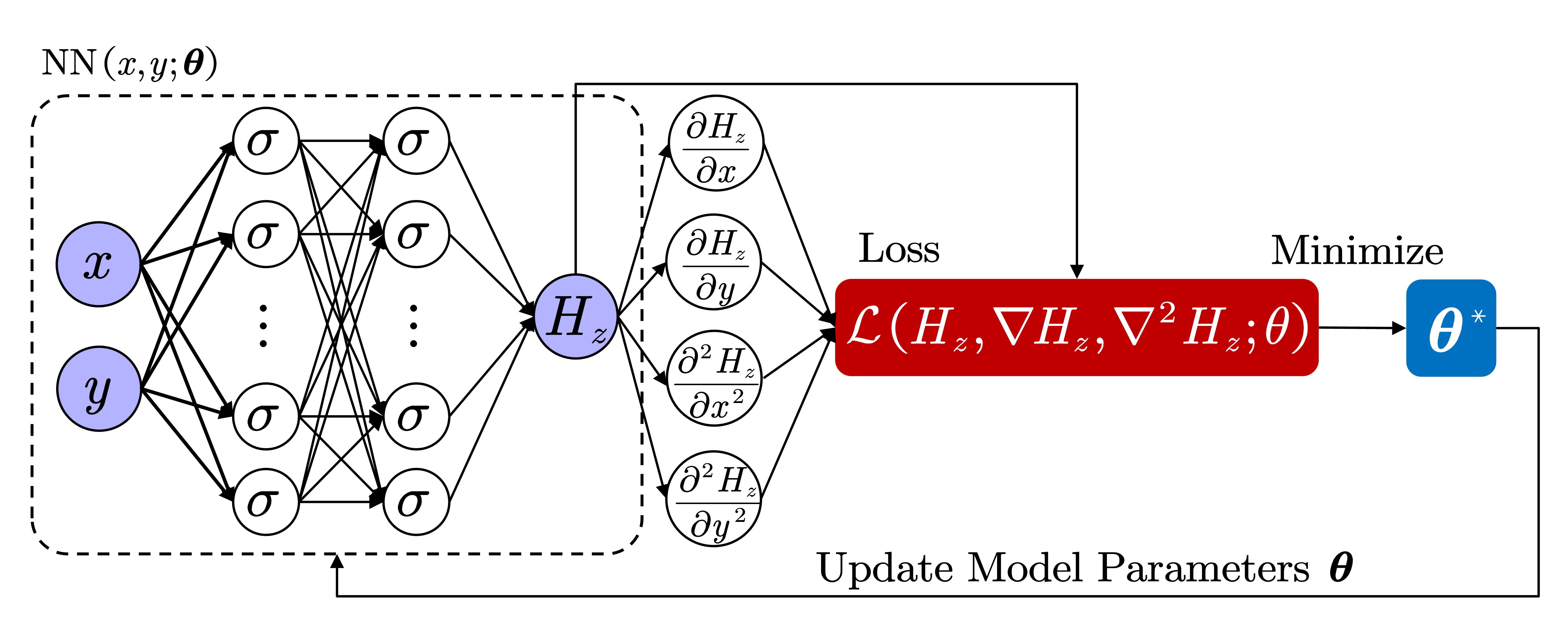
A Deep Learning Framework for Solving Rectangular Waveguide Problems
Xiaolin Hu, Nicholas E. Buri, APMC 2020 (Oral) |
- We employ Physics Informed Neural Networks (PINNs) to solve rectangular waveguide problems.
- We successfully apply PINNs to the task of solving electric and magnetic fields, which can be described by partial differential equations (PDEs).
- We also show the applicability of the framework for predicting the unknown parameters such as wavenumber.
-
APMC 2019
Capacity Estimation of MIMO Systems via Support Vector Regression
Xiaolin Hu, Nicholas E. Buri, APMC 2019 (Oral) -
APMC 2020
Multiple Signal DoA Estimation with Unknown Electromagnetic Coupling using Gaussian Process
Qifeng Wang, Nicholas E. Buris, Xiaolin Hu, APMC 2020
🚍 Others
ICIP 2021
3D Grid Transformation Network For Point Cloud Completion
Xiaobao Deng, Xiaolin Hu, Nicholas E. Buris, Ping An, Yilei Chen, ICIP 2021- Wavelength-tunable Q-switched fiber laser based on a 45 tilted fiber grating
Xiaolin Hu, Zhijun Yan, Qianqian Huang, Chuanhang Zou, Tianxing Wang, Chengbo Mou, Opto-Electronic Engineering 2018
🎖 Honors and Awards
- 2022.10 First-class Scholarship, Renmin University of China, Beijing, China
- 2021.10 Second-class Scholarship, Renmin University of China, Beijing, China
- 2019.12 Second Prize, China Post-graduate Mathematical Contest in Modeling, China
- 2019.12 Third Prize in Shanghai, China Graduate Electronics Design Contest, Shanghai, China
- 2018.07 Provincial Outstanding Graduates, Shanghai, China. (top 5% of graduating students)
- 2018.07 President Scholarship, Shanghai University. (top 15 of 4900 graduating students)
- 2017.11 First prize in Shanghai, National Undergraduate Electronics Design Contest, Shanghai, China
📖 Educations
- 2021.09 - Present, Ph.D. in Artificial Intelligence, Renmin University of China, Beijing.
- 2018.09 - 2021.07, M.S. in Communication and Information System, Shanghai Univeristy, Shanghai.
- 2014.09 - 2018.07, B.S. in Communication Engineering, Shanghai Univeristy, Shanghai.
💻 Internships
- 2020.10 - 2021.02, OPPO Research Institute, Intelligent Communication Lab, Shanghai.